Navigating Your AI Journey as a CX Leader
The AI landscape has massive potential, lots of shiny objects, lots of immature vendors, and lots of pressure to move fast. It’s FOMO like never before.
It’s indisputable that AI has a ton of potential. The transformative impact it brings could be huge for your business.
That’s why you need a crystal-clear strategy when it comes to using AI.
In the CX industry, we’ve learned from past experience that jumping too quickly into the deep end can create unintended consequences.
Ineffective chatbots, IVR mazes, uncoordinated multi-channel, integration bottlenecks, desktop experiences that no one can navigate, dead-end experiences, disjointed and unconnected processes.
The list goes on and on.
When this happens, customers hate the experience we provide. These problems are everywhere, and in every company. We’ve been able to fight through some of these challenges over time, but time and budget constraints have impacted our strategy, our results, and limited customer satisfaction with our brands and service.
In the dynamic landscape of customer experience leadership, one concept stands out as a wise guiding principle when it comes to adopting AI and mitigating risk.
"Crawl, Walk, Run" .
This mantra encapsulates the recommended approach for CX leaders venturing into the world of AI, ensuring a gradual but effective immersion into this transformative technology.
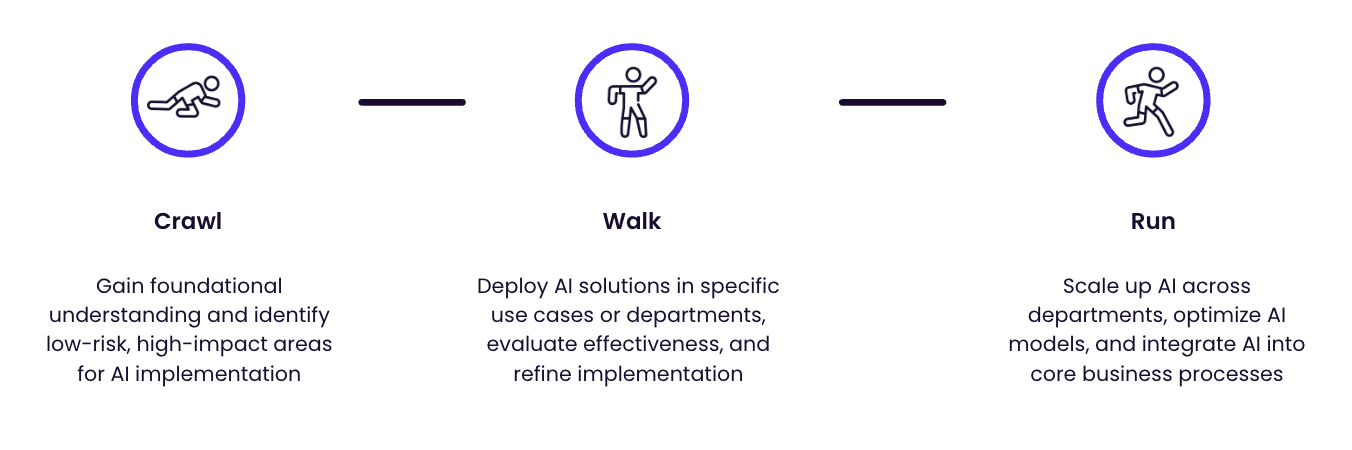
Crawl: The first steps in your AI journey
The "crawl" phase is your initial foray into the realm of AI. It's time to educate yourself and your team about the possibilities that AI brings to the CX table. Picture this phase as your AI orientation—a time to grasp the fundamentals. The "crawl" phase sets the stage for your AI journey as a CX leader. It's a phase of exploration, education, planning, communication, and evaluation.
Education is key
Start by educating yourself on AI. Understand the core concepts, terminology, and the diverse array of AI technologies available. As a CX leader, it's crucial to be familiar with the different types of AI, including generative models, Large Language Models (LLMs), and more.
Recommendations:
- Play with Generative AI: Get used to leveraging generative AI with ChatGPT to uncover information and perform research
- Transform and re-write content: Leverage ChatGPT to rewrite content, break processes down into steps. Improve writing, and fill in gaps. This will provide an eye-opening experience and highlight both opportunities and challenges in generative AI technology. Nothing beats first-hand experience.
Suggested reading list:
- Humanizing AI - Is AI Good or Evil?
- Humanizing AI - Where Humans Remain in Control
- A 7-Step Guide to Implementing AI in Consumer-Focused Businesses
- AI Agents - From Software to Autonomous Software
- How ChatGPT turned generative AI into an “anything tool”
- What are GPT Agents? A deep dive into the AI interface of the future
- GPT-4 Can Use Tools Now—That’s a Big Deal
Suggested podcasts:
Who's in the game?
In this phase, it's important to identify the key players in the AI landscape. Who are the companies, startups, and experts making significant strides in AI for CX?
There are five main types of AI technology providers today:
- Hardware & infrastructure providers: These industry giants offer the foundational hardware and infrastructure essential for running AI models. Examples: Nvidia, Intel, Amazon, AMD, and Google.
- Foundational model makers: These are the trailblazers in building core AI functionality engines. Other tech entities often license their models to enhance their own offerings. Examples: Stability AI, Meta, Google, OpenAI, Microsoft, NVIDIA, and Amazon.
- Software vendors powered by AI: These platforms cater to specific business use cases, seamlessly integrating AI models—often from third parties—into their technology. Their primary aim is to drive specific business outcomes. Examples: Zingtree, HubSpot, Notion, IBM Watson, and Adobe Sensei.
- LLM consumers with general data: These companies innovate by leveraging both open-source and commercially available Large Language Models (LLMs) to craft applications. Examples: GPT-based chatbots, Grammarly, and Replika.
- LLM consumers with proprietary data: These are specialized firms that design bespoke applications, often by layering proprietary data or uniquely training LLMs for targeted outcomes. Examples: BenevolentAI, Primer, and Talkspace.
Developers will typically investigate using technology from firms in category 1 for core internal AI projects and model development and training, while business and operations teams will typically focus on leveraging vendors in category 2 & 3 to solve specific business problems through commercially delivered SaaS solutions.
What's at stake?
Consider what's at stake for your organization: your brand reputation, your customer satisfaction, and, ultimately, revenue retention.
The "crawl" phase allows you to evaluate the potential benefits and risks associated with AI adoption.
Your primary job in the crawl phase is to de-risk projects to ensure manageable learning, execution, and demonstrable results, all with minimal risk.
You’ll also be testing your organization and team’s readiness to explore AI-related change. Even if you’re ready, is your legal and information security team ready to support you? Do you need to help them build new competencies? Do you need to help them understand how their crawl phase and your crawl phase can work together?
You need to spearhead the crawl phase plan for all potential stakeholders, from agents, to administrators, to operations, to marketers, to executives, to legal and compliance…the list goes on.
Crawl phase planning enables you to surface fears and start addressing them across your organization. Over time, this builds the confidence and competencies you need for even the most basic AI projects.
The urgency and FOMO factor
CX leaders often feel the urgency to embrace AI.
Guess what? Your executive team and your board feel the same pressure. The fear of missing out (FOMO) on AI-driven advantages can be a compelling motivator. The rapid pace of technological advancements means that waiting too long can result in falling behind your competitors. There’s some truth to this, which is often behind any pressure you’re feeling to deploy AI.
AI is about doing what you currently do better, faster, and more seamlessly. It's not a new business strategy, at least when you’re starting out in the crawl or walk phases. To see transformative impacts from AI—and to create FOMO in your competitors—you need to get to the run phase of AI deployments. But you can’t run until you first crawl and then learn to walk. Shortcuts don’t end well on this journey.
We’re emphasizing this point strongly because it’s vital to articulate this to your executive team. You need to get buy-in for your AI strategy as a journey, starting slowly and eventually building momentum.
Compliance and ethical considerations
In this age of data privacy and ethical AI, compliance should be a paramount concern.
Ensure that your AI initiatives align with legal and ethical guidelines. A misstep here can lead to serious consequences for your organization, which is why you need to consider it during the crawl phase. Getting this right will mitigate risks and build momentum for future projects.
Commit to becoming an expert
At this stage, you’re already among the elite 1% who recognize the potential of AI in CX.
Commit to becoming an expert in AI for your industry. Attend conferences, webinars, and courses. Stay updated on the latest trends and research.
AI will impact CX more than many other functions in your business, meaning you’ll need to be an AI expert for the long haul. Commit now to your multi-year learning strategy.
Here’s the good news: you don’t have to go to business school for this. You don’t have to feel overwhelmed.
Start with an article once a week or a podcast every other week. Build a network of peers also interested in deploying AI in CX. Work with your team and internal stakeholders on their fears. As you learn, help educate them.
Becoming an expert in AI—and deploying it effectively in your organization—is a marathon, not a sprint.
Small but consistent learning over time is the best way to grow your own AI expertise.
Planning for skills and team development
The "crawl" phase isn't just about your personal journey. It's also an opportunity to plan for your team's development.
Start by assessing your team's current skill set and identify gaps that need to be filled. AI is scary for a lot of team members. They’re concerned it will take away their jobs, and they probably don’t yet understand that AI needs humans in the loop to make the biggest impacts.
Introducing AI shouldn’t feel threatening, although it will induce some changes in your team. But you can get ahead of those changes by identifying the skills your team will need to enable them to work well with AI, then proactively training them in those areas.
Education enables change, buy-in, and participation from your team members.
The role of data
Data is the lifeblood of AI. In the "crawl" phase, take stock of your data assets. Understand what data you have, where it's stored, and its quality. Clean, reliable data is essential for successful AI implementations.
In this phase, don’t worry about connecting data sources. It’s better to start by understanding where data, knowledge, and systems of processes and actions are located in your environment. Typically this will help you uncover areas of suboptimal process and actions, or areas that lack process or actions entirely.
Setting clear goals
Define clear and achievable goals for your AI initiatives. Whether it's improving response times, personalizing customer interactions, or automating routine tasks, having well-defined objectives will improve the odds of your success with AI.
During the crawl phase, don’t dream too big. Descope your projects. Improve one thing and learn from it, then do one more thing.
Sometimes these “surface” level AI deployments will seem unremarkable at first. Helping an agent troubleshoot a problem, writing a better email response, or automating a single process doesn’t feel revolutionary.
But each of these small improvements builds incrementally on one another.
Babies learn to lift their heads. Then they learn to roll over. Then they learn to crawl.
Every step builds the foundation and experience that enables the next step, and the results compound over time. Also, remember many of these small first projects are new (by design) and have the potential to fail, but because they are small these failures will also be unremarkable—but they’ll provide great learning experiences for future projects.